A new road safety testing simulation multiplies adverse events, so commercially available driverless vehicles can get on the road faster.
One of the major roadblocks for fully autonomous vehicles is effective safety testing software. Testing autonomous cars on actual roads is incredibly time consuming, expensive and potentially hazardous to other drivers.
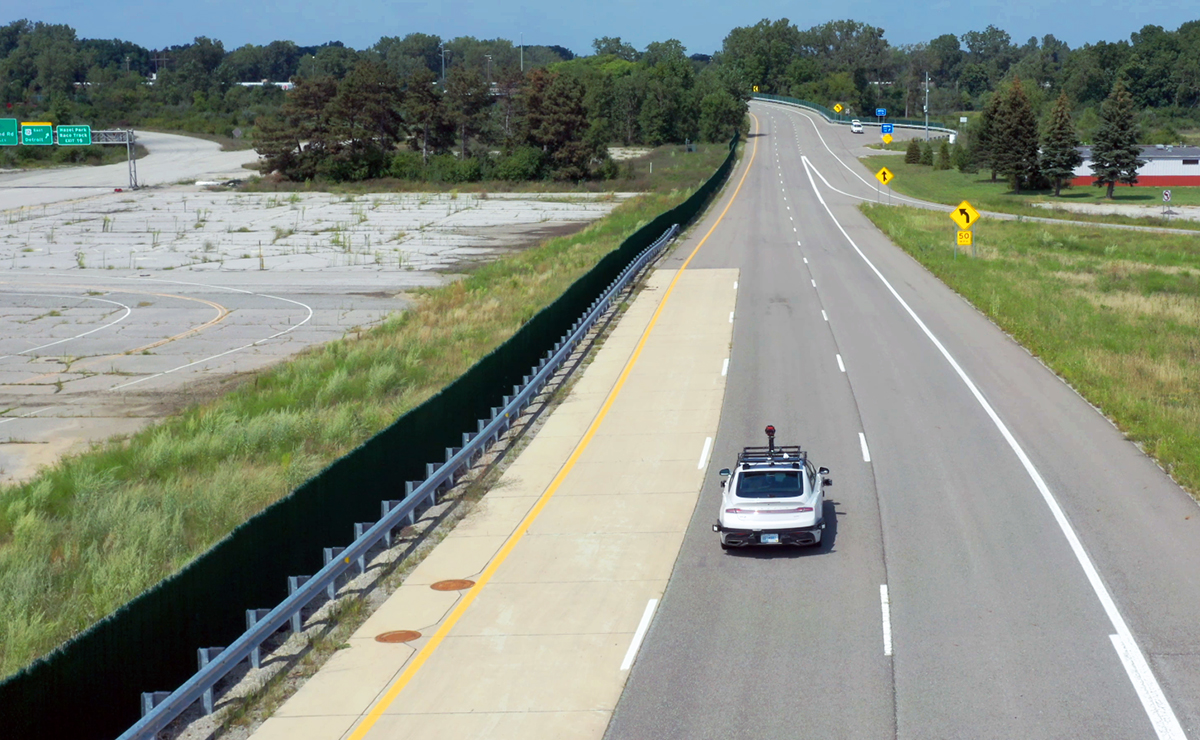
The researchers tested their enhanced autonomous-vehicle safety testing AI in an augmented-reality environment that included simulated background vehicles and a real car and test track.
Because of the limits to obtaining real-world data, the accuracy of artificial intelligence (AI) simulation testing — which is less dangerous but relies on the input of real-world data for accuracy — is also constrained, explains Shuo Feng, an alumnus from Tsinghua University’s Department of Automation.
To get enough data on real-world hazards for AI simulations, each physical test vehicle needs to drive for hundreds of millions to hundreds of billions of kilometres, which isn’t practical.
But now, in a paper published in the journal Nature1 in March 2023, Feng and a team from the University of Michigan in Ann Arbor, US, report on a method to boost the speed of autonomous vehicle safety testing by up to 100,000-fold.
Calling in reinforcements
Their new system addresses the issue of the rarity of ‘safety-critical incidents’ — such as near misses, pre-collision events or crashes. It does this by reinforcing real-world driving data with ‘densified data’, a modelling technique that plots highly likely outcomes to increase the number of observations in a dataset, explains Feng. These tweaks ultimately led to faster and more accurate decision-making by their test vehicle in augmented-reality driving scenarios.
The researchers tested their AI on a real mid-size luxury saloon or sedan car called a Lincoln MKZ hybrid, produced by American car manufacturer Ford. The vehicle was modified into a ‘level 4 autonomous vehicle’ — which means it doesn’t require human attention or input — using a popular open-source driving system called Autoware.
“We demonstrated the effectiveness of our approach by testing on both a 4km highway and urban test tracks in the United States. The tests used an augmented-reality environment that included simulated background vehicles, alongside the actual physical road infrastructure and a real autonomous test vehicle,” explains Feng.
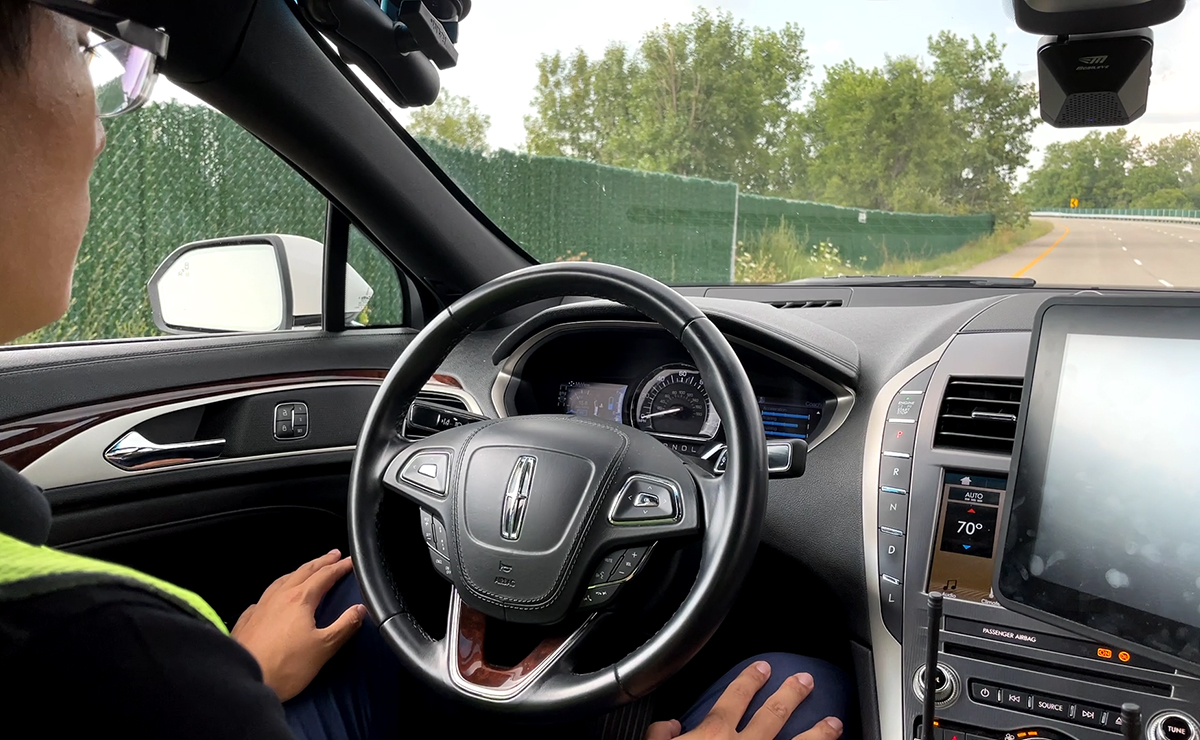
The new AI was tested using a real mid-size luxury saloon or sedan car fitted with a popular open-source driving system called Autoware.
AIs assessing other AIs?
In that environment, they simulated safety-critical events using their AI and evaluated crash types and crash severities in addition to crash rates.
Their results showed that their ‘densified data’ AI testing system can accelerate the simulated safety evaluation process by many orders of magnitude — landing at between 1,000 and 100,000 times faster than real-world driving tests. But it remains to be seen how much national testing authorities will accept this in place of real-world test.
The study could have implications for more than just cars, providing a means to improve safety simulations for everything from autonomous drones and planes to more android type robots. “The study is advancing AI-based methods to validate AI-based systems. It’s essentially AI against AI,” Feng point out.
But for cars, he hopes this advance will provide a potentially affordable and timely path forward for safety testing.
“This type of research will be critical for manufacturers, governments, and third-party agencies, such as insurance agencies, who will ultimately decide whether AVs are ready for large-scale deployment,” explains Feng. “It will also be important for customers, who will need to have more confidence in the autonomous vehicles.”
Reference
1. Feng, S., Sun, H., Yan, X. et al. Dense reinforcement learning for safety validation of autonomous vehicles Nature 615, 620–627 (2023) Doi: 10.1038/s41586-023-05732-2
Editor: Guo Lili