The implementation of neuromorphic computing system based on RRAM electronic synapses
On May 12,Professor He Qian and Huaqiang Wu’s research group at the Institute of Microelectronics, published a research article entitled "Face Classification using Electronic Synapses " in Nature Communications. This work presented an analogue non-volatile resistive memory (as electronic synapse) with CMOS compatible process. The device shows bidirectional continuous conductance weight modulation characteristics which could simulate synapse behavior nicely. Grey-scale face classification is experimentally demonstrated using an integrated 1024-cell array with parallel online training. The energy consumption within the analogue synapses for each iteration is 1,000x lower compared to an implementation using Intel Xeon Phi processor with off-chip memory.
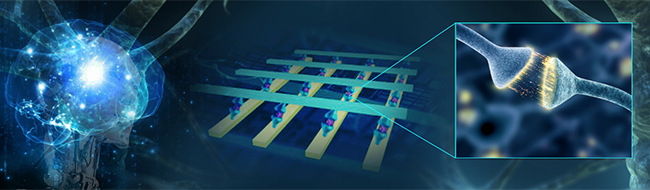
Image of Brain-Inspired Computing based on RRAM array
Compared with current hardware approach, human brain is more effective to complete cognitive tasks. To design a neuromorphic chip that emulates the fundamental network structure of brain, a large amount of synapses which act as the connection of neurons should be integrated. RRAM is a promising high-efficiency electronic device to realize the plasticity of synapse and is capable of reaching ultra-high integration density. Professor Qian and Wu’s research group, collaborated with researchers from Stanford University led by Prof. Philip Wong, have succeeded in fabricating a 1024-cell electronic synapse array with RRAM device and demonstrated a neural network prototype to realize face image recognition based on Yale Face database.
The fabricated 1024-cell electronic synapse array is of remarkable and reliable bidirectional analog switching behavior; the device stack uses CMOS fab-friendly material. This paves the way for building a neuromorphic hardware system on a scale similar to the human brain.
RRAM (or memristor) devices were conceived by electrical engineer Leon Chua in his 1971 paper "Memristor--the Missing Circuit Element" and experimentally discovered by Hewlett Packard in 2006. Conductance of RRAM device could be tuned for strong or weaken continuously by applying an appropriate voltage pulse. This is in agreement with the modulation of synapse weight. To achieve the goal of fabricating a large and reliable electronic synapse array, the Tsinghua research group optimized the device stacks. The conductive metal oxide layer is inserted to improve the bidirectional analog switching performance. The resistive switching layer is changed into a laminate structure. The 1T1R basic cell structure is adopted to suppress the sneak path and improve the conductance modulation further.
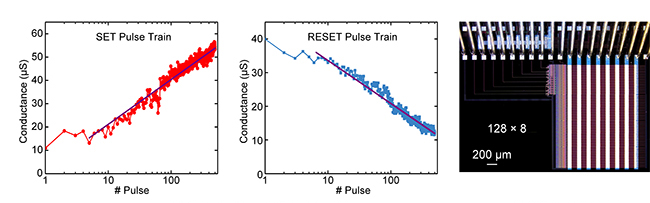
Typical bidirectional continuous conductance tuning performance under identical pulse train and the micrograph of a fabricated array.
The research team also claimed that they demonstrated a fully connected artificial neural network to recognize face images from Yale Face database. The electronic synapse based system finally realizes similar recognition accuracy and tremendous energy saving than traditional Von Neumann computing system. “This experimental prototype is a convincing example for the application of neuromorphic computing system based on RRAM electronic synapses array,” Huaqiang told us.
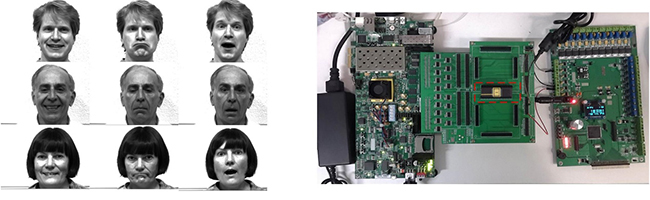
The face images from Yale Face database and the setup of test platform
Ph.D. student Peng Yao is the first author and Prof. Huaqiang Wu is the corresponding author of this work. This research was supported by Beijing Innovation Center for Future Chip, the National Key Research and Development Program and the National Natural Science Foundation of China.
Related link: https://www.nature.com/articles/ncomms15199